Technological advancements enable fast and high-resolution hyperspectral imaging for the research and clinical markets.
HOD FINKELSTEIN, RON R. NISSIM AND MARK J. HSU, TRUTAG TECHNOLOGIES INC.
Hyperspectral imaging (HSI) provides images with numerous spectral bands over a continuous spectral range, enabling analysis of complex scenes that contain both spectral and spatial information. These imagers tend to be expensive and often require external processing of the large amounts of data captured in each scan. Recent advances in tunable filter elements combined with increased computational power of embedded processors have resulted in a fully autonomous battery-operated and small form-factor imager. The imager can capture a full hyperspectral data cube and distill it to the information of interest in less than five seconds and at a price point that previously had been unattainable.
Much of the spectral information in nature is broadband — the sun’s blackbody emission spectrum, the reflectance spectrum of flowers and the absorption spectrum of blood are just a few examples. In order to minimize the amount of sensory information that needs to be processed by our brains, nature developed optical sensors in the form of cone-shaped cells in our retinas. These have broad absorption spectra in the blue, green and red regions of the spectrum.
Solid-state image sensors, such as the CCD and the CMOS sensor, are inherently monochromatic. They convert the photons that are absorbed within the depletion region of their PN junctions into charge, regardless of their wavelength. In order to mimic our color perception, broadband polymer color filters are deposited on top of these junctions to create a color filter array (CFA). Similarly to our cone cells, these filters transmit in the blue, green and red regions of the spectrum. Pixel arrays coated with such CFAs are commonly known as RGB sensors.
In reality, significant information can be gleaned from fine spectral features. These features may arise naturally or be custom designed. High-resolution spectral information in nature is used to identify atomic and molecular emission and absorption lines and subtle spectral shifts because of metabolic changes in tissue, as well as to recognize plants, animals or various pathologies.
In recent years, various man-made optical labels have been introduced and widely used to tag biological targets. These include fluorescent molecules and quantum dots, each having a characteristic spectrum. Observing these objects and processes with RGB sensors is akin to a farsighted person trying to read a book: The person may be able to read the chapter titles, but not their contents. Hyperspectral imagers address the need to collect optical information with both high spatial and spectral resolutions, thus enabling us to acquire a more accurate picture of biological objects and processes.
Spatial and spectral resolution
In order to understand how HSIs work, it is helpful to understand two related sensing technologies. Spectrometers analyze the spectral content of light without acquiring information in the spatial distribution of these spectra. As such, they work well for analyzing bulk homogenous materials. Such devices are quite simple and recently have been integrated into cellphones.
Multispectral imagers acquire two-dimensional images at several spectral bands, usually by applying a CFA with narrower passbands. These devices trade spatial resolution for spectral resolution. For example, a sensor with a 16-color CFA will have 16 times lower spatial resolution than the underlying monochromatic sensor. Such devices offer very fast acquisition times at a relatively low cost, but are inadequate when finer spectral information is needed or when spatial resolution cannot be compromised.
True hyperspectral imaging provides a nearly continuous spectrum concurrently with high spatial resolution. Traditional RGB or multispectral imagers deliver “images” that are constructs containing XY location and a digital number corresponding to the collected number of photoelectrons per location. HSI replaces these with hyperspectral data cubes. These are three-dimensional structures that contain spatial information in two axes and spectral information in the third. In other words, each pixel in a data cube contains as much information as a complete measurement from a spectrometer. As a result, hyperspectral data cubes can become very large and are typically more than a gigabyte.
Acquiring these data cubes can be achieved in three ways. Whisk broom imagers use a traditional spectrometer that can capture the spectrum of a single spot and raster scan the field of view. Such devices require a long time to acquire a data cube, and this acquisition time scales with the field of view.
Push broom imagers sample a linear section of the field of view onto a grating. The imagers project the resulting spectra onto a traditional two-dimensional image sensor so that one of its axes corresponds to a physical dimension and the other to wavelength. Such imagers require the grating to scan across the field of view. Typically, this makes such systems expensive and mechanically sensitive. Their acquisition time scales as the dimensions of the scanned axis.
Staring or snapshot imagers do not require mechanical scanning. Instead, they employ a tunable filter element that selects one or more spectral bands for each exposure, generating a complete data cube. The acquisition time does not depend on the number of pixels, but rather on the number of spectral bands being acquired. These imagers are especially attractive for applications requiring high spatial and spectral resolutions with tunable spectral ranges and a small form factor.
Various devices have been developed for snapshot HSI. These typically utilize wavelength-dependent interference effects to pass or block narrow bands of light. Acousto-optical and liquid-crystal tunable filters use acoustic or electronic transducers to modulate the birefringence of a medium, thereby selecting optical bands to be transmitted. The devices achieve relatively narrow passbands, but suffer from a limited operating range. And scanning the whole visible range with high resolution has not yet been demonstrated commercially.
Fabry-Perot interferometers (FPIs) operate by placing two mirrors parallel to each other. By controlling the reflectivity of the mirrors, high-finesse filtering can be achieved. At any given intermirror spacing, only a few interference orders are transmitted through the device (typically fewer than three in regular operating conditions). Therefore, the gap needs to be scanned in order to cover a spectral range. Robust piezoelectric-activated tunable FPIs covering the whole visible range as well as other spectral bands with resolutions on the order of 10-nm full width half maximum (FWHM) have been demonstrated by VTT Technical Research Centre of Finland. Such devices can be placed either in the focal plane or in the Fourier plane of imaging systems. But each of these configurations trades off system-level performance parameters.
One of the main challenges in building a tunable FPI device for high-resolution HSI arises from the requirements for mirror flatness and coplanarity. A collimated beam entering an FPI with high-reflectivity silver mirrors will undergo an average of 10 reflections before being transmitted out. Because the intermirror gap determines the wavelengths to be transmitted, any variability in this gap across the mirror will cause different wavelengths to be transmitted. In the case of placement in the focal plane, the effect of this variability will be a variance in the transmitted wavelengths as a function of position on the FPI aperture. If the FPI is positioned in the Fourier plane, the result will be a spectral averaging (broadening). The high reflectivity of the mirrors, which is required for achieving high spectral finesse, amplifies the impact of the variability with both focal plane and Fourier plane placement.
Intermirror gap variability can either be caused by surface roughness of the mirrors, by nonflatness or by imperfect coplanarity. A typical FPI aperture is about 10 mm. In order to achieve high finesse with a line width on the order of 10 nm FWHM, the combination of surface roughness, mirror flatness and coplanarity must be on the order of 1 nm, or seven orders of magnitude smaller than the diameter.
This must be achieved in real time while scanning the FPI at rates of tens to hundreds of gaps per second.
A recently announced imager (Figure 1) demonstrates the performance potential of FPI-powered imagers. The mass-manufacturable imager integrates a TruTag tunable FPI with an off-the-shelf CMOS image sensor and custom control and processing electronics. It delivers 2.3 megapixel hyperspectral data cubes in 400 spectral bands with a FWHM of approximately 15 nm in less than five seconds.
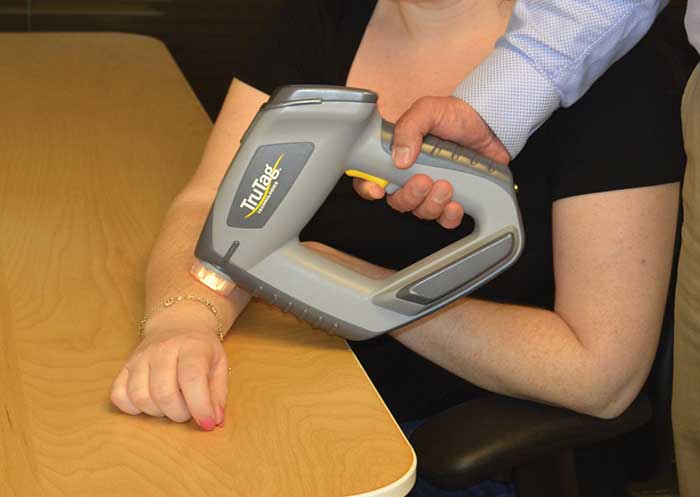
Figure 1. TruTag Model 4100 handheld hyperspectral imager collects optical information with high spatial and spectral resolutions. Courtesy of TruTag Technologies.
The battery-operated handheld device also addresses another problem in hyperspectral imaging: the large data sets being generated. With more than a gigabyte of data being generated in just a few seconds, efficiently moving, processing and storing the data becomes critical. The imager enables fast and power-efficient processing through implementation of algorithms to identify features of interest and minimize the data set close to the sensing node.
Applications in the life sciences
Important information on the structure and state of biological samples can be gleaned from label-free HSI (Figure 2). In microscopy, multiplexed spectral imaging allows tracking a larger number of targets while colocalizing these targets. Recently, a technique in which 120 fluorescent labels were used concurrently was demonstrated. In order to also be able to localize the labeled objects, a high-resolution camera is required.
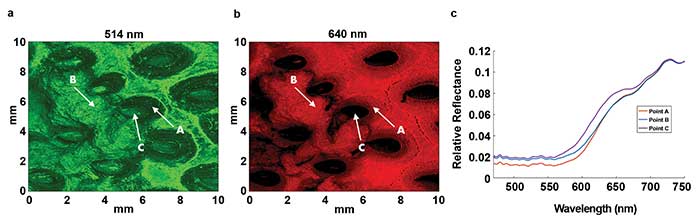
Figure 2. Hyperspectral images (a and b) can be used to identify and localize ripe (point A) and overripe (point B) regions in a strawberry. The characteristic reflectance spectra (c) of these regions as well as of a seed (point C) can be used for both research and quality control. Courtesy of TruTag Technologies.
The recent permission from the FDA to market the Philips Intellisite Pathology Solution (PIPS) for whole slide imaging opens the door for HSI to be applied in digital pathology. Scanning systems such as PIPS allow for maintaining continuous focusing. Snapshot or stepping cameras with a large field of view will reduce the number of images to be stitched, stored and processed; deliver more information; and reduce the overall cost of such systems. The number of color channels in state-of-the-art digital pathology systems such as the PerkinElmer Vectra Polaris currently stands at around 10. Spectral resolution is achieved using a filter wheel. As the number of spectral channels increases, filter wheels will become less suitable and HSI is likely to take their place.
In diagnostic applications, HSI has been used in such fields as oncology, dermatology and ophthalmology. HSI has also been used in cancer diagnostics to detect and localize protein biomarkers, to grade cancers by hyperspectral analysis of tumors and to identify tumor boundaries. In addition, hyperspectral imaging has been used to classify melanomas versus common nevi with high sensitivity and specificity and to monitor changes in skin over time using spectral information.
Ophthalmological applications include retinal HSI to monitor oxygenation levels and retinal ischemia, which can be caused by diabetes. Age-related macular degeneration can be diagnosed by hyperspectrally monitoring the oxidative state of cytochrome c during oxidative stress. More broadly, as a spectrally calibrated high-resolution imaging modality, HSI can facilitate telemedicine by allowing the transmittal of detailed medical images without losing spectral or spatial information.
Hyperspectral imaging is still a nascent field. Challenges remain to the cost-effective production of tunable filter elements, to high frame-rate capture and processing of data cubes, and to extracting actionable information from the large data sets. As this technology becomes more ubiquitous, we are bound to see it widely implemented not only in the research lab but also in diagnostics and medical imaging.
Meet the authors
Hod Finkelstein, Ph.D., is chief technology officer at TruTag Technologies Inc. in Emeryville, Calif.; email: [email protected]. Ron Nissim, Ph.D., is systems engineer at TruTag; email: [email protected]. Mark J. Hsu, Ph.D., is systems engineering manager at TruTag; email:[email protected].
Case study: Comparing spectral imaging in dermatology
Much of the early diagnostics in the field of dermatology is based on visual inspection of the skin. A large number of tissue disorders, such as erythema, skin cancer and vitiligo, manifest themselves as changes in color or pigmentation. Analysis of these conditions can be made by combining absolute reflectance measurements with measurement of characteristic spatial features to compare suspect features to benign ones and monitor spatio-spectral images over time.
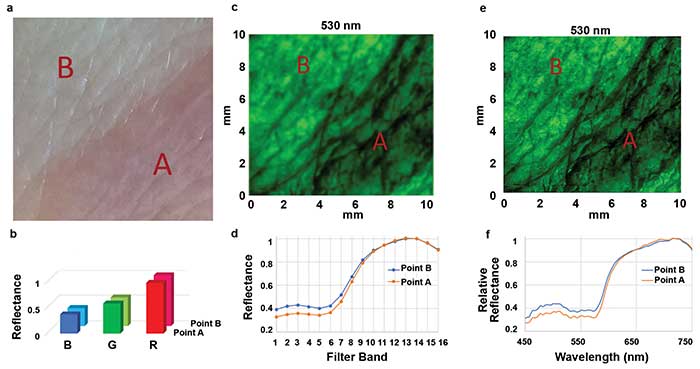
In this example of spectral imaging in dermatology, a skin condition known as granuloma annulare (point A) can be compared with healthy skin (point B) using a standard RGB camera (a). The resulting spectral resolution (b) is extremely poor. A 16-color CFA multispectral imager delivers 1/16th the spatial resolution (c) with finer spectral resolution (d). The 530-nm spectral slice captured by a TruTag 4100 hyperspectral imager provides megapixel spatial resolution (e) with fine spectral resolution (f), allowing a detailed examination of spectral information as well as associated spatial features. Courtesy of TruTag Technologies.
Digital imaging provides the opportunity to electronically store this information for future use. However, RGB cameras only provide very broad spectral resolutions and are often uncalibrated. Consequently, images captured using RGB cameras cannot discern fine spectral features. If subsequent images are captured using different cameras, these cannot be reliably compared. Multispectral cameras are available with 16 or 25 spectral bands. These typically capture images with high efficiency, but trade off spatial resolution. Hyperspectral imagers can provide both high spatial and spectral resolution. This comes at the price of lower light throughput, because at a given moment, only a few spectral bands are imaged. Light flux on the sample can be reduced significantly by placing the tunable filter element on the illumination arm of the imaging system.