AI Chip Lends Credence and Application to Analog in-Memory Computing
Relying on an analog circuit, a new AI chip from imec and GlobalFoundries can perform in-memory computations with an energy efficiency 10 to 100 times greater than those that use a traditional digital accelerator. The chip, called AnIA (for “Analog Inference Accelerator”) is optimized to perform deep neural network calculations on in-memory computing hardware in the analog domain.
Developers built the AnIA on GlobalFoundries’ 22FDX semiconductor platform, which features high-level energy efficiency. Characterization tests demonstrated power efficiency reached 2900 tera operations per second per watt (TOPS/W). The degree of efficiency is such that pattern recognition in tiny sensors and low power edge devices, those machine learning in data centers typically power, can now be performed on the accelerator.
A new design of the conventional architecture of digital computer processors and memory units enhances and streamlines neural network functionality. Digital computers have traditionally featured a disconnect between memory and processor, posing a problem of efficiency in certain operations requiring the use of large data volumes. These operations have necessitated use of a correspondingly large volume of data elements, which the system must collect from memory storage.
Known as the von Neumann bottleneck, this limitation can outweigh the actual time it takes digital computers to perform computations. This is especially common in neural networks — relying on large vector matrix applications to effectively process and classify images and information across applications, yet requiring a significant amount of energy to perform complex computations.
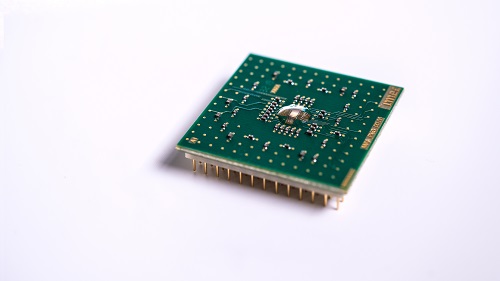
AnIA test chip mounted on the PCB used for measurement and characterization. Courtesy of imec.
Importantly, neural networks can also deliver accurate results when vector matrix multiplications are performed with a lower precision on analog technology. With cheap, accessible, ultralow power at a premium, imec and its industrial partners in its industrial affiliation machine learning program, which includes GlobalFoundries, configured a new architecture that performs analog computation in SRAM cells, eliminating the von Neumann bottleneck.
“The successful tape-out of AnIA marks an important step forward toward validation of Analog in Memory Computing (AiMC),” said Diederik Verkest, program director for machine learning at imec.
“In imec’s machine learning program, we tune existing and emerging memory devices to optimize them for analog in-memory computation. These promising results encourage us to further develop this technology, with the ambition to evolve toward 10,000 TOPS/W."
Verkest said there may be some concern about accuracy with an analog circuit, as opposed to a digital circuit, given common variables like noise. However, the overall performance level of the AnIA, paired with energy efficiency, places the accelerator on the cutting edge of its technology.
“From our perspective, this was a milestone in the machine learning program. We wanted to convince the research partners that an analog computation for something that is typically done in the digital domain can actually work and have the same accuracy you can achieve with digital computations,” Verkest said.
Another prominent feature involves device security. Some of the common potential issues the singularity of the chip eliminates are latency, unwanted data access (privacy), and external network reliability.
“All data remains on the device, rather than relying on network infrastructure and the cloud,” Verkest said. The ability to ensure data security has potential to expand the variety of applications and machines that may opt to implement the chip.
“This test chip is a critical step forward in demonstrating to the industry how 22FDX can significantly reduce the power consumption of energy-intensive AI and machine learning applications,” said Hiren Majmudar, vice president of product management for computing and wired infrastructure at GlobalFoundries.
“The analog compute is a phenomenal frontier because it allows you to manage the reduction of the data moment between compute and memory elements. We expect that analog computebased silicon will be hitting production at the end of this year, early next year. In terms of mass market deployment, we anticipate analog compute getting into mass market certainly no later than 2022 — but it could potentially happen sooner than that.”
/Buyers_Guide/Imec/c22187