The combination of multiple images from various sensors results in the effective detection of distance as well as potential hazards for self-driving automobiles.
Pablo García-Gómez, Jordi Riu, and Santiago Royo, Beamagine SL
Imaging lidar sensors are one of the primary components in systems used for perception tasks in autonomous vehicles. The 3D data generated by lidar while a vehicle is in motion is considered useful because such data is the result of measuring real-time physical occurrences. These include events such as the time delay between two laser pulses (in the case of the pulsed time-of-flight technique) or the Doppler shift of the returned signal (in the frequency-modulated continuous-wave approach).
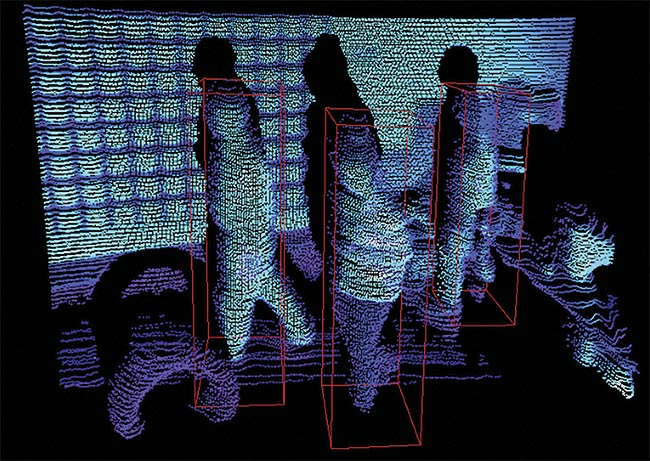
Preinstalled perception software for automated human detection based on 3D point cloud analysis. Courtesy of Beamagine.
Unlike with other 3D techniques — such as stereovision — with lidar, post-processing is unnecessary because collecting 3D data does not depend on the use of software algorithms. Lidar also carries its own illumination source, which eliminates dependency on external illumination. However — even though lidar data has been proved to be reliable throughout the automotive industry — for increased reliability, the modality must be combined with other sensing technologies, whether it is being used in an autonomous vehicle or a perception system for critical applications. These vehicles and systems can’t allow false detections. Second and even third sources of information that record the same field of view (FOV) congruently help to reduce dependency on a specific technology, and they minimize the chances of perception failure.
Congruent data fusion
The sensors most often combined with lidar are radar and cameras, which together can collect spectral information from any band, from the visible wavelengths to the longwave infrared (LWIR). Data fusion techniques are used to combine the information from all the sensors. The two main approaches are early and late data fusion. The early approach fuses the image data before perception, while the late approach fuses the information at the object level after perception. The early fusion approach has been shown to provide a higher level of reliability1.
However, the early option must more precisely fuse the images received from the various imaging modes. Parallax issues can cause the 3D data from the lidar and the images from the cameras to be less coincident in the whole field of view for all distances, which can compromise the robustness of the entire system. Parallax is common and in some cases impossible to avoid, especially when the sensors are spread across the surface of the vehicle. The varying perspectives of the FOV can be difficult for the data fusion software to manage.
Also, the sensor system’s manufacturer must provide a lifetime guarantee of the mechanical alignment of the product after calibration. If the system receives even a small amount of distorted information, parallax misalignment between sensors results, and a new calibration process is required.
A recently developed combined-imaging system includes lidar sensors and uses congruent data fusion — integrating data from all distances simultaneously — as one of its main features (Figure 1). This functionality allows accurate and parallax-free fusion at all distances. The standard version of the sensor incorporates two complementary types of cameras: RGB for the visible spectrum and LWIR to collect thermal data.
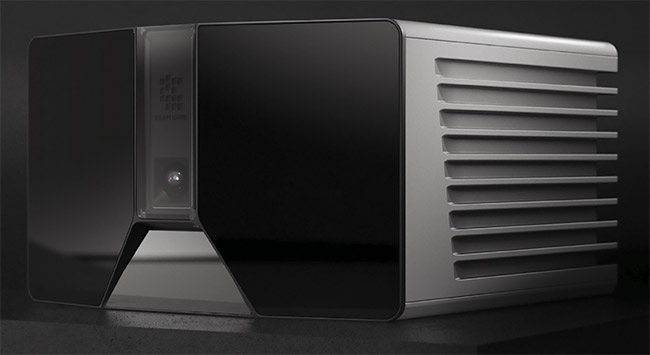
Figure 1. The L3CAM incorporates triple sensor integration. The lateral windows correspond to the lidar apertures, the central top area integrates an RGB camera, and the central bottom window includes a thermal camera. Courtesy of Beamagine.
However, cameras that image at other wavelengths — such as near-IR, shortwave-IR, midwave-IR, polarimetric, and even multispectral cameras — can also be used. The cameras are integrated within the same casing, so mechanical alignment is guaranteed.
The information gathered from the three aligned sensors is free of parallax issues, and it can be managed via the user’s own perception software algorithms. A comparison of detached versus integrated cameras in sensor systems is shown in the table.
Figures 2 and 3 show examples of various imaging modes.
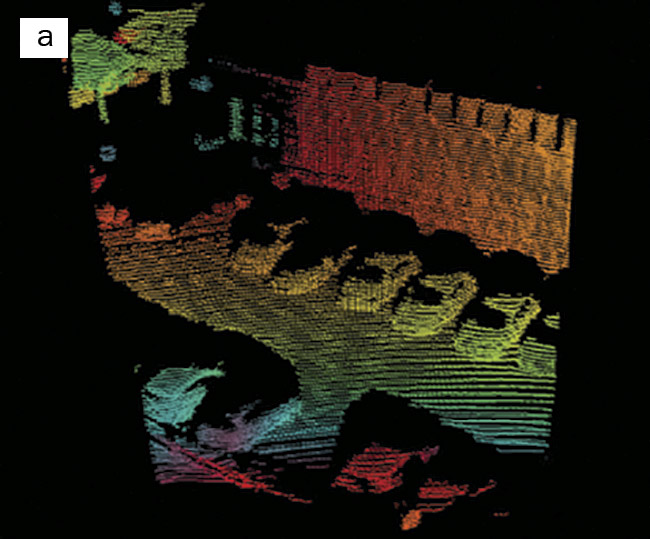
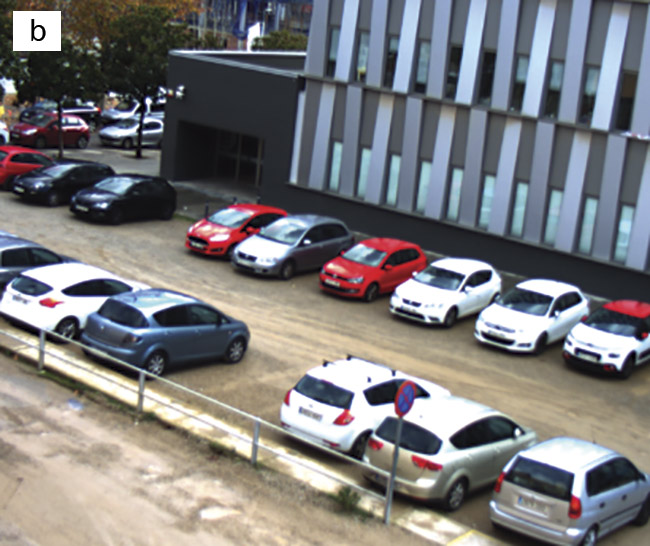
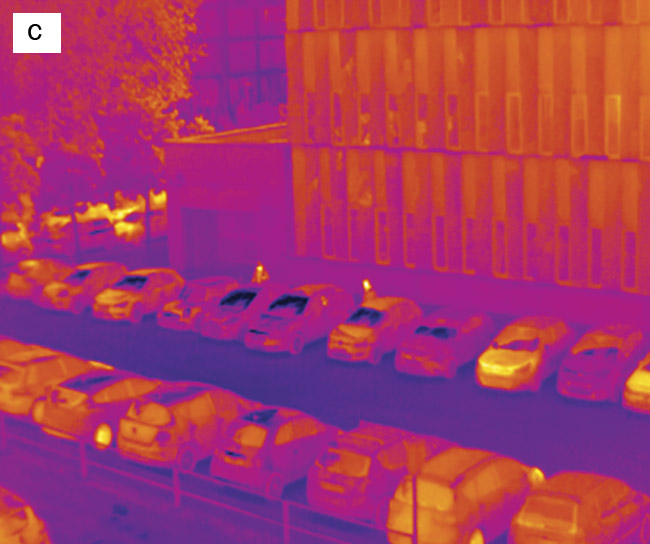
Figure 2. A 3D point cloud image (a), an RGB image (b), and a thermal image (c). Courtesy of Beamagine.
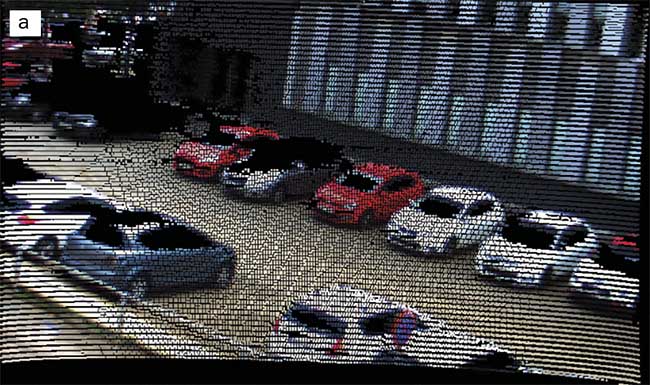
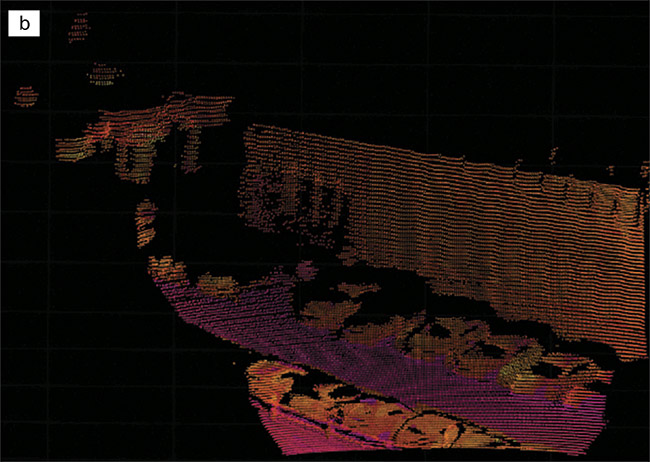
Figure 3. 3D image data overlapped by RGB data (a). 3D image data overlapped by thermal data (b). Courtesy of Beamagine.
Reducing false alarm rates
The combination of complementary imaging modes can effectively reduce the false alarm rates that are typical when AI perception software is in use. The use of redundant and complementary data sources allows the user to find more ways to face a perception challenge2. An example of this can be found in the perception AI for automated pedestrian detection. Humans can be detected within the FOV of an RGB camera using resources such as the real-time object detection system and algorithm YOLO (You Only Look Once).
In ideal working conditions, such a tool can achieve >90% effectiveness when detecting humans (lead image of story). However, when the conditions are less than ideal (e.g., at night or in bad weather), the false alarm rate rapidly increases. Changes in illumination, requirements for small cross-section object detection, or the presence of bad weather can make a combined imaging system useless because of frequent false alarms.
In challenging conditions, sensors with complementary failure modes can be used to provide redundancy in object detection, as well as tolerance, to help avoid collecting unreliable data. For instance, thermal cameras complement RGB detection because they provide better performance than RGB cameras during nighttime and in foggy conditions. And the lidar can determine the size of objects, so eliminating the uncertainty derived from calculating the aspect ratio at various distances becomes possible. This three-sensor combination dramatically reduces the false alarm rate and improves the robustness of the AI software, enabling reliable training of AI perception.
Solid-state scanning
The market is focused on solid-state devices in lidar applications because of their lack of large moving elements. Large mechanics such as motors or rotating heads that intervene during the generation of optical images are critical elements that can fail when installed on a vehicle. It has been demonstrated that MEMS scanners are less sensitive to vibrations than motorized systems due to their resonance frequencies, which are higher than the typical vehicle vibration frequencies3. These make a sensor system much more tolerant to the real-world environment in which a vehicle would be operated.
One key aspect of a lidar sensor that is designed for fast-moving vehicles such as automobiles is the capability to detect any object on the road. To accomplish this task, the resolution of the point cloud at the vertical axis becomes crucial. Typically, any object larger than 10 cm can damage the bumper, so the perception of the vehicle must be able to detect the object in time to change course.
The combination of a decent vertical FOV with a sufficient vertical angular resolution and range is not easy to achieve. The resulting point cloud has to contain a large number of lines, typically around 200. As long as the y-axis resolution of the integrated sensor system is not limited to a fixed number of emitters or receivers, this resolution can be configured by the user.
However, with lidar comes trade-offs, one of which is that this increased resolution on the y-axis translates to a lower frame rate. In the case of Beamagine’s L3CAM configuration, which contains 200 horizontal lines (Figure 4), the frame rate would be limited to 10 fps.
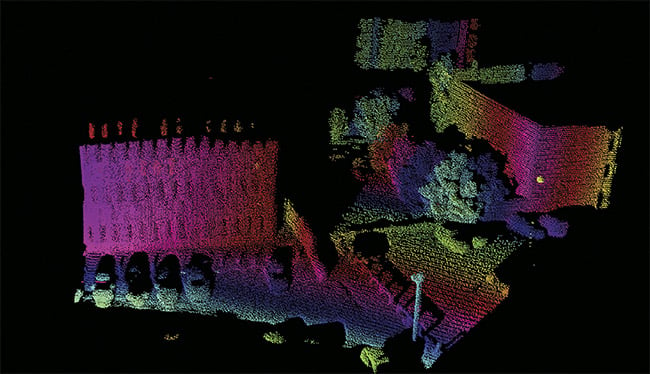
Figure 4. A 600- × 200-pixel single-frame point cloud image. Courtesy of Beamagine.
Applications
Vehicle robotics is one main application of such a system, especially when the robotics operate outdoors and need the capacity for long-range detection of obstacles. The automotive industry is another target of this technology, as is satellite docking.
A comparison of the specifications of detached and integrated
cameras in sensor systems
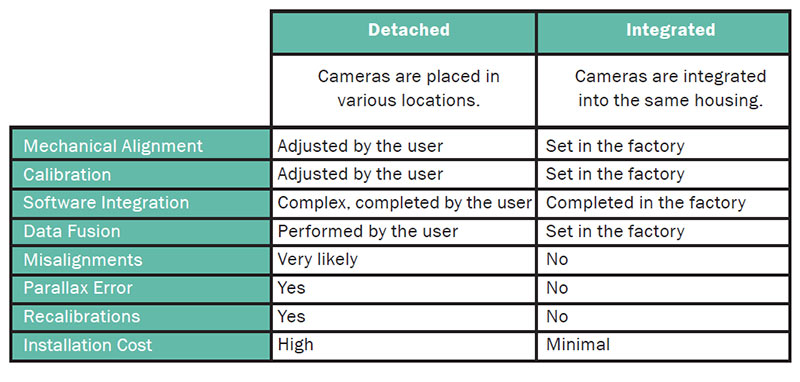
However, any platform that requires sensing — including vessels, cranes, trains, drones, and off-road vehicles — is compatible with these systems. Other applications include static installations for security and surveillance, such as intrusion detection, perimeter protection, monitoring of unattended control centers, detection of humans on rail tracks, and crowd analytics.
Meet the authors
Pablo García-Gómez is a doctoral student in optical engineering at the Technical University of Catalonia, where he received his master’s degree in physics engineering. He is completing his doctoral work related to lidar image fusion at Beamagine while he contributes to various research projects at the university.
Jordi Riu is CEO and co-founder of Beamagine. He has a master’s degree in electronics and a doctorate in optical engineering from the Technical University of Catalonia. His doctorate work, which generated several patents, centered on solid-state lidar imaging based on MEMS scanners. He has been working in hardware development for lidar imaging for the last 10 years.
Santiago Royo is co-founder of Beamagine, where he is vice president of business development. He is also co-founder of
the photonics-based spinoff companies SnellOptics and ObsTech SpA. Royo holds 17 patents, 11 of which are licensed to four different companies. He has authored papers for over 50 peer-reviewed publications.
References
1. T.Y. Lim et al. (December 2019). Radar and camera early fusion for vehicle detection in advanced driver assistance systems. Machine Learning for Autonomous Driving workshop at the 33rd Conference on Neural Information Processing Systems (NeurIPS 2019).
2. R. Hebbalaguppe et al. (2016). Reduction of false alarms triggered by spiders/cobwebs in surveillance camera networks. IEEE International Conference on Image Processing (ICIP), Phoenix, www.doi.org/10.1109/icip.2016.7532496.
3. J. Iannacci (2015). Reliability of MEMS: a perspective on failure mechanisms, improvement solutions and best practices at development level. Displays, Vol. 37,
pp. 62-71.